Artificial intelligence in the context of evaluation
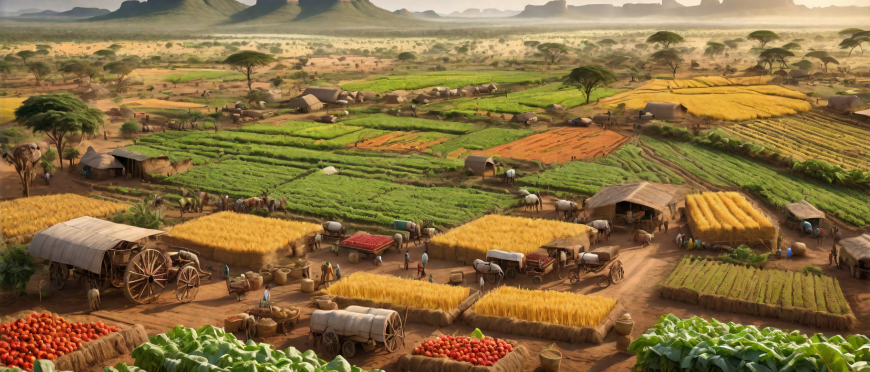
Dear all,
As we enter the age of Artificial Intelligence (AI), how can we as evaluators take advantage of this development in our professional activities? Do you have any AI models to share, and what types of activities can they be applied to? How can we harness this technology effectively?
In addition, what new skills should evaluators develop to remain competitive and meet the growing expectations of the field?
I would really appreciate your experiences and advice and thank you in advance for sharing your knowledge on this fascinating subject.
Muriel KOUAM
This discussion is now closed. Please contact info@evalforward.org for any further information.
Ram Khanal
Advisor Community of Evaluator (COE) NepalI have not used in evaluation per se but used for literature review and other purposes. I am aware this will be a useful tool but not sure whether the information available or used by chat gpt is adequately represent the context and information for the developing countries like Nepal. I do hope - colleagues who have used the tool will be in position to share their experience.
Sada Hussain Shah Syed
EvaluatorI have been conducting simple thematic analysis in Chat-Gpt. I found it simple and supportive as compared to nVivo.
Dushyant Mahadik
Assistant Professor National Institute of Technology RourkelaI have used clustering algorithms to segregate satellite data and tag different regions with the crop grown in each region. The biggest challenge I have faced in applying AI is the availability of reliable data from field. Typical evaluation surveys are not sufficient to implement AI. In my opinion, large scale 'open' datasets are necessary before AI gets meaningful.
Khalid NADIFI
CEO Waman consulingWe re using AI algorithms for leak detection in distribution networks.
That include rémotion sensing and analysis
Muriel Kouam
MEAL Manager OXFAMHello everyone,
As the various contributions testify, AI is unavoidable at this time, especially for MEL communities. So thank you all for your valuable contributions!
For those interested in taking action, Ann Murray will be running a master class in April on qualitative data analysis, reporting and more using CHATGPT, Perplexity.ai and claude.ai. In short, how to make the most of these AIs in a practical evaluation context. There is a charge for this course, and here is the registration link Ann-Murray Brown 🇯🇲🇳🇱’s Post
agusdin pulungan
President WAMTII agree with you Lendsey.
Chat GPT is still not enough to give an appropriate answer. In some cases is very limited with numeric data.
Best,
ABLAYE GAYE
PRÉSIDENT Président comité local thies de RACINES (Réseau des Acteurs et Initiatives et Économiques , Écologiques et Solidaires)Good evening,
I can give a concrete example from our agriculture initiative in southern Senegal, where we are using drones to assess agroecological practices.
Thanks to AI, we can analyze these practices in depth: the camera-equipped drone we used collects valuable crop data while flying over the fields. This approach enables an accurate assessment of farming activities, with an emphasis on agroecology. By observing family farms from the air, drones provide crucial information on crops, irrigation, soil management and many other aspects. This has enabled us to make an accurate assessment of the various farming activities in the southern zone of Senegal, with its emphasis on agroecology. Drones offer an aerial perspective that can confirm whether farming practices are really in line with this approach. Drones therefore play an essential role in assessing family farms and promoting agroecology.
There are also highly effective applications that use algorithms for programming. For example, in Senegal, on the farms I was following, the times of fertilization and treatment on the farms are programmed by a very efficient algorithmic application that even tells you what type of fertilization you need to do, and then during the harvesting phase it lets you know with a precise assessment the possibilities of harvest quantity and all the expenses to be used during the harvesting period. They send reminders to our computers and cell phones. It's really handy for optimizing farming practices and improving efficiency. That's why I've enrolled in a virtual computer university (web gaming application development) in Senegal in agronomy since 2022! In a few years' time, when I've acquired the necessary knowledge, I'll be able to participate as an agronomist in the development of agricultural applications.
It's really exciting to see how technology can support sustainable agriculture!
ABLAYE GAYE
PRÉSIDENT Président comité local thies de RACINES (Réseau des Acteurs et Initiatives et Économiques , Écologiques et Solidaires)Advances in artificial intelligence offer many opportunities to improve our professional activities, including in the field of evaluation. Here are some commonly used AI models and their potential applications:
1. Machine learning: This model enables AI to learn from data and take decisions or make predictions. It can be used in risk assessment, performance prediction, survey data analysis, etc.
2. Natural language processing: This model enables AI to understand and analyse human language. It can be used to analyse comments, extract information from documents, automatically classify responses, etc.
3. Computer vision: This model enables AI to understand and analyse images and videos. It can be used for satellite image analysis, anomaly detection, quality inspection, etc.
To take effective advantage of these advances, here are a few tips:
1. Understand your needs: Identify the areas of your business where AI can add value. Identify repetitive tasks, data collection and analysis processes, and areas where AI can help make more informed decisions.
2. Acquire skills: Develop your skills in machine learning, natural language processing and computer vision. There are many online resources, training courses and communities to help you acquire these skills.
3. Collaborate with AI experts: Work with AI experts to develop solutions tailored to your specific needs. They can help you build AI models, collect and analyse the necessary data, and interpret the results.
Lindsey Moore
DevelopMetricsDear Colleagues,
Thank you, Muriel, for initiating this insightful discussion, and I greatly appreciate all the valuable contributions from everyone.
At DevelopMetrics, we work a lot with USAID and other donors building Large Language Models to analyze evaluations and other documents for decision-making. One caution that I would like to share is that we have conducted multiple benchmarking studies and found that generalized AI like ChatGPT is not effective at understanding the nuances of development terminology. For example, if you ask ChatGPT which interventions have historically been most successful at empowering women in Pakistan, you're relying on a model that is trained on all evidence on the internet and built based on a Silicon Valley data architecture - in other words, you're perpetuating existing biases. This is why domain-specific models that are vetted by technical experts are so important.
I hope that's helpful.
Best regards,
Lindsey
Lindsey Moore
CEO & Founder
+1 646-593-4568
www.developmetrics.com
Anna Maria Augustyn
International consultantDear Muriel and Colleagues,
Thank you for the questions and insights. I’d like to share some experiences I made while working on a large database (thousands of projects) from which a portfolio had to be extracted for the impact evaluation. The methodology utilized machine learning algorithms, a branch of AI.
The approach was two-fold: 1) a machine learning algorithm was developed by the experts, and 2) a semi-manual search was performed. In the first case, the portfolio turned out to be smaller than expected, but the projects were very precise and on the topic of interest. Yet, the portfolio was too small to make robust statistics out of it. In the second approach, the portfolio was much bigger but many projects had to be removed from the dataset as they were marginally correspondent to the topic of interest. An expert guidance was needed to define the keywords and refine the portfolio and a programming expert to develop a customized application. Subsequent activities proved to be very fruitful with using language-based processing of the projects and available evidence on the web (web-scrapping, incl. social media).
The following methodology challenges could be observed:
To conclude, I found AI very useful there where large datasets and portfolios were available for the analysis, and where the web data was abundant. It can help greatly, but at the same time requires a good quality assurance as well as dedicated expertise.
I am concerned about the privacy and security in using AI. It is already difficult to harmonize the approach in the international cooperation, especially with projects from different donors and legal systems at the international and national or even institutional levels. But we should give it a try!
Best wishes,
Anna Maria Augustyn
Maya Zahabi
Country M&E Officer UNFAODear Muriel,
Thank you for raising such an interesting topic. I have much to say but ill try to keep it brief.
i would like to raise a few points i find very interesting and i hope you do too. There is a lack of consensus amongst practitioners on the definition of AI. There are some broad and lose definitions, but not a clear definition used by all. By some definitions, a calculator could be defined as AI, and yet, most do not consider it as such.
Another point id like to raise, is AI is built on learning models, the ability to learn and apply. By saying this, we agree that there must be a learning memory. In our field this becomes tricky as most data we use is classified as highly sensitive, are unclear/undefined on data sharing, or for some the ownership of data is unclear, this is especially true for organizations/agencies that work with governments.
I would also like to emphasize, there is currently no regulation for AI, which means it carries some substantial risk. This brings us to some of the ethical considerations for introducing the field to AI:
We should additionally ask ourselves, what are we really hoping to get from AI and is it absolutely necessary?
To date, there have been many successful attempts by organizations to adopt some forms of AI safely, i think there is much to learn from those experiences as well, here are some links below:
https://www.fao.org/agroinformatics/news/news-detail/fao--ai-and-digital-tools-for-climate-resilient-agri-food-systems--on-the-spotlight-at-the-science-and-innovation-forum-2023/en
https://www.fao.org/vietnam/news/detail-events/en/c/1187477/
https://dref.ifrc.org/fba/
https://jetson.unhcr.org
https://hungermap.wfp.org
Additionally, here are some articles that i found helpful to support critical thinking and i hope they prove helpful to you too.
https://www.humanitarianstudies.no/resource/data-sharing-between-humanitarian-organisations-and-donors/
https://gh.bmj.com/content/7/Suppl_8/e007249
https://onezero.medium.com/are-aid-agencies-abetting-surveillance-humanitarianism-5bc2b5a78ff6
https://journals.sagepub.com/doi/full/10.1177/2056305119863146
https://international-review.icrc.org/sites/default/files/reviews-pdf/2022-02/biometric-data-flows-and-unintended-consequences-of-counterterrorism-916.pdf
https://journals.sagepub.com/doi/full/10.1177/2056305119863146#fn1-2056305119863146
https://www.thenewhumanitarian.org/news/2019/02/05/un-palantir-deal-data-mining-protection-concerns-wfp
All the best to you in your brave endeavor!
Pantaleon Shoki
Dear Aurelie,
My name is Pantaleon Shoki, and I have the honor of serving as the Executive Secretary of the Tanzania Evaluation Association (TanEA). I would like to share exciting developments in the realm of evaluation, particularly regarding the integration of Artificial Intelligence (AI) in our field.
The African Evaluation Association (AfrEA), a premier pan-African non-profit dedicated to enhancing evaluation practices continent-wide, is hosting an international conference. I am thrilled to announce that I will be presenting a paper titled "Revolutionizing Monitoring, Evaluation, and Learning (MEL) Systems Through Artificial Intelligence" at this prestigious event. The conference, themed “Technology and Innovation in Evaluation Practice in Africa: The Last Nail on the Coffin of Participatory Approaches?” promises to be a groundbreaking forum for professionals in our field.
As I prepare for this significant opportunity, I am in the process of seeking sponsorship to support my participation in the conference. Additionally, I am looking for avenues for future publication and partnership possibilities that align with our shared interest in leveraging AI for evaluation. To this end, I would be grateful for your assistance in identifying potential funding opportunities that could sponsor my paper presentation and mark the beginning of a promising collaboration in AI for Evaluation.
For colleagues and industry professionals interested in this innovative conference, I am pleased to share the registration link https://afrea.org/conference/ . Your support in exploring sponsorship possibilities would not only contribute to the success of my presentation but also pave the way for future collaborative endeavors in this dynamic field.
I look forward to the possibility of working together to advance the integration of AI in evaluation practices.
Warm regards,
Pantaleon Shoki, Executive Secretary of the Tanzania Evaluation Association
Aurelie Larmoyer
Senior Evaluation officer WFPDear Muriel,
I agree A.I. brings so much potential for evaluation, and many questions all at once!
In the Office of Evaluation of WFP, as we have looked to increase our ability to be more responsive to colleagues’ needs for evidence, the recent advancements in artificial intelligence (A.I.) came as an obvious solution to explore. Therefore, I am happy to share some of the experience and thoughts we have accumulated as we have started connecting with this field.
Our starting point for looking into A.I. was recognizing that we were limited in our capacity to make the most of the wealth of knowledge contained across our evaluations, to address our colleagues’ learning needs. This was mainly because manually locating and extracting evidence on a given topic of interest, to synthesize or summarize it for them, take so much time and efforts.
So, we are working on developing an A.I. powered solution to automate evidence search using Natural Language Processing (NLP) tools, allowing to query our evidence with questions in natural language, a little like we do in any search engine on the web. Then, making the most of recent technology leaps in the field of generative A.I, such as Chat GPT, the solution could also deliver text that is newly generated from the extracted text passages, such as summaries of insights.
We also expect that automating text retrieval will have additional benefits, such as helping to tag documents automatically and more systematically than humans, to support analytics and reporting; and as that Ai will also give an opportunity to direct relevant evidence directly to audiences based on their function, interests and location, just like Spotify or Netflix do.
As we manage to have a solution that offers a good performance in the search results it offers, we hope it may then be replicable to serve other similar needs.
Beyond these uses that we are specifically exploring in the WFP Office of Evaluation, I see other benefits of A.I. to evaluations, such as:
I hope these inputs are useful, and look forward to hearing the experiences of others, as we are all learning as we go, and this is indeed full of promises, risks and surely moves us out of our comfort zones.
Best
Aurelie
Cristian Maneiro
Associate Maestral InternationalGreetings colleagues,
Thank you, Muriel, for bringing up this topic, and I appreciate all the contributors. I believe that AI holds promising features for evaluators, and it's crucial for us to be aware of them. Personally, the prospect of conducting fast and interactive quantitative analysis without the need for expertise in code-based software (e.g., R or Python) would be a game-changer for professionals like myself with backgrounds in human sciences.
Additionally, the capability of summarizing extensive raw texts, such as interviews or focus group discussion transcripts, and facilitating accurate analysis of key points, has the potential to save a significant amount of time. However, it's essential to highlight that the evaluator's experience, prior knowledge of the field, insights from stakeholders, and a sense of the evaluation's purpose will continue to be crucial and valued.
Moreover, ethical dilemmas and decisions on how to present results won't be solved by AI, no matter how powerful it becomes.
I would love to see examples of AI used in both quantitative and qualitative approaches.
Vicente Plata
ConsultantDear Muriel,
Thank you for this very challenging and intelligent topic you pose to the group!
I will share my thoughts about the second question. With the development of AI we all (evaluators as well as all other workers) are challenged to provide relevant value through intelligent contributions to the task. So, from my perspective, we will have to sharpen our contributions to an evaluation process that possibly will be at least partially carried on by AI.
I will be paying attention to the contributions of our colleagues on AI models, types of activities to be applied to, and experiences.
Best regards
Vicente
Senior evaluation consultant
Lal - Manavado
Consultant Independent analyst/synthesistGreetings!
First of all, prudence and common demands that one should carefully ascertain the following before any new technology is applied to a given field, in this case, evaluation:
Cheers!
Lal Manavado.
Mohammed Al-Mussaabi
AI has proven to be a powerful assistant for professional evaluators. However, it is essential to recognize AI as an assistant rather than a standalone solution. Some AI users tend to overly rely on it without applying critical thinking and human judgment, leading to subpar results. When used appropriately, AI can greatly enhance the evaluation process by automating tasks, analyzing large volumes of data, and providing valuable insights. It can assist evaluators in data collection, organization, analysis, and visualization, saving time and improving efficiency. AI's capabilities in text analysis and predictive analytics enable evaluators to uncover patterns, sentiments, and trends, supporting more accurate recommendations and decision-making. Nevertheless, it is crucial for evaluators to exercise caution and maintain a balanced approach. Human expertise, critical thinking, and contextual understanding are still vital in interpreting AI-generated insights and ensuring their validity. Evaluators must filter and validate AI-generated outputs, considering the limitations and potential biases of the algorithms.
Adéléké Oguniyi
MERL ExpertAs we embrace the era of Artificial Intelligence (AI), evaluators have a unique opportunity to leverage this technology to enhance their professional activities in several ways:
To harness AI effectively, evaluators should consider the following strategies:
In addition to technical proficiency in AI, evaluators should cultivate a range of complementary skills to remain competitive and meet the evolving expectations of the field: